Logistic regression as MLE solution
Written on August 3rd , 2020 by Sergei Semenov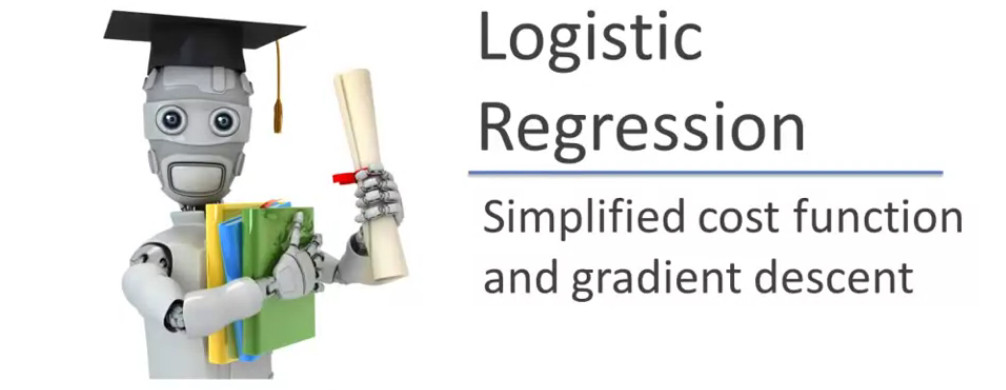
Introduction
A friend of mine tried to tell me, that no one in the world understands, how Artificial neural networks (ANNs) actually work. Moreover, he said, that people somehow select parameters/features/architectures, train network and find the best ANN, but still people don’t understand how it works. Even 1 layer ANN network has a lot of magic inside.
I am not 100% agree with this statement. In this post, I want to show, that 1 layer ANN for binary classification is nothing more than maximum likelihood estimator.
Logistic regression cost function
In Machine Learning class at Coursera the following logistic regression cost function was introduced:
\[\operatorname{cost}\left(h_{\theta}(x), y\right)= -y * \log \left(h_{\theta}(x)\right) - (1-y)\log \left(1-h_{\theta}(x)\right)\]It was unclear for me, where this function comes from come. This expression looked very familiar to me. Let’s try to derive it using classical estimation theory.
Model selection
Assume that, we have a set of features, denoted as \(\theta_{i}\), and training samples \(D = \{x[m], y[m]\}_{m=1}^{M}\)
Outputs (\(y[m]\)) can take only binary values. In our training set the exact number is \(M_0\) and \(M_1\) for values \(0\) and \(1\) respectively. \(x[m]\) is a series of input vectors (features).
We will use a logistic regression model:
\[P(Y=1\mid\theta) = h_{\theta}(x)= \frac{1}{1 + exp(-\theta^{T}x)}\]It means, that:
\[P(Y=0\mid\theta) = 1 - h_{\theta}(x) = \frac{exp(-\theta^{T}x)}{1 + exp(-\theta^{T}x)}\]MLE in action
Out goal is to find \(\theta\), that suit features the best. Let’s calculate MLE for a given training set \(D\):
\[L(\theta : D) = \prod_{m=1}^{M_1}{\hat{P}(y[m]=1\mid x)} \prod_{m=1}^{M_0}{\hat{P}(y[m]=0\mid x)}\]or in logarithmic form:
\[l(\theta : D) = \sum_{m=1}^{M_1}{\log\hat{P}(y[m]=1\mid\theta)} + \sum_{m=1}^{M_0}{\log\left(1 - \hat{P}(y[m]=1\mid\theta)\right)}= \\ = \sum_{m=1}^{M_1}\log(h_{\theta}(x)) + \sum_{m=1}^{M_0}\log(1 - h_{\theta}(x))\]It is easy to make next step. We can extend the first expression over all possible \(M\) by introducing indicator functions:
\[l(\theta : D) = \sum_{m=1}^{M}y[m]\log(h_{\theta}(x[m])) + \sum_{m=1}^{M}(1 - y[m])\log(1 - h_{\theta}(x[m])) = \\ = \sum_{m=1}^{M} \big( y[m]\log(h_{\theta}(x[m]) + (1 - y[m])\log(1 - h_{\theta}(x[m]))\big)\]MLE solution doesn’t change if we scale by \(M\). Also, instead of maximizing likelihood, we can minimize the negative likelihood. And the cost function, which we want to minimize, now is:
\[J(\theta) = \frac{-l(\theta : D)}{M}= \frac{1}{M} \sum_{m=1}^{M} \big(- y[m]\log(h_{\theta}(x[m]) - (1 - y[m])\log(1 - h_{\theta}(x[m]))\big)\]Conclusions
The probability theory is extremely helpful to understand many topics in ML
Last update:03 August 2020