Belief Propagation in Clique Trees
Written on July 5th, 2020 by Sergei Semenov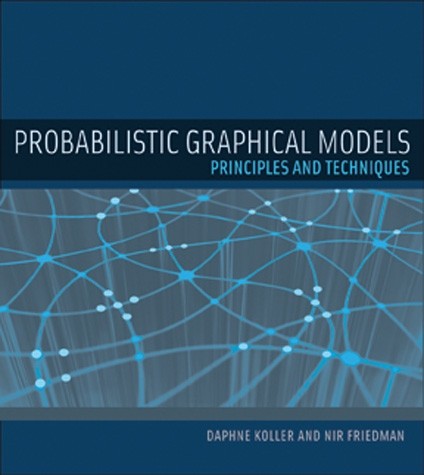
BP recall: Calibration property
Reference: Properties of Belief Propagation
When BP is running, the messages are transferred from one Cluster to another. With new message, every Cluster updates it’s belief
\[\beta_{i}\left(\boldsymbol{C}_{i}\right)=\psi_{i} \times \prod_{k \in \mathcal{N}_{i}} \delta_{k \rightarrow i}\]There is a theorem, that convergence leads to calibration:
\[\sum_{C_{i}-S_{i, j}} \beta_{i}\left(C_{i}\right)=\sum_{C_{j}-S_{i, j}} \beta_{j}\left(C_{j}\right)\]Calibration property implies that product of messages on both sides of the link is the marginal over the cluster beliefs:
\[\mu_{i, j}\left(S_{i, j}\right)= \delta_{j \rightarrow i}\left(S_{i, j}\right) \delta_{i \rightarrow j}\left(S_{i, j}\right)=\sum_{C_{j}-S_{i, j}} \beta_{j}\left(C_{j}\right)\]The \(\mu_{i, j}\) is called as “Sepset belief”.
BTW If we multiply beliefs over all clusters and divide it to all messages, we can get unnormalized joint distribution:
\[\frac{\prod_{i} \beta_{i}}{\prod_{i, j} \mu_{i, j}}=\frac{\prod_{i} \psi_{i} \prod_{j \in \mathcal{N}_{i}} \delta_{j \rightarrow i}}{\prod_{i, j} \delta_{i \rightarrow j}}= \prod \psi_{i}=\tilde{P}_{\Phi}\left(X_{1}, \ldots, X_{n}\right)\]For details one can refer to Properties of Belief Propagation
BP in Clique trees
The one special application of running BP is message passing in clique trees or Junction tree algorithm. In this case, BP works faster and gets convergence to the exact inference. Moreover, the resulting beliefs are guaranteed to be correct marginals.
Q: What is the difference with Variable Elimination?
A: The variable elimination calculates marginals only for desired cluster. BP calculates unnormalized marginals for all clusters simultaneously
Q: drawbacks?
A: yes, we need to apply proper scheduling for messages (start from leaves)
Q: other benefits?
A: yes, it can simplify computations using evidence. How? Run BP. Take any cluster, that has required variables and do marginalization. The output will be unnormalized measurements
If we have a new evidence Z, find a cluster that has variables from Z and do computations only in this cluster. Calculate the \(P_{\psi}(X \mid Z) = \frac {\widetilde{P}_{\psi}(X, Z)}{\widetilde{P}_{\psi}(X)} = \frac {\widetilde{P}_{\psi}(X, Z)}{\sum_{Z}\widetilde{P}_{\psi}(X \mid Z)}\)
Reference: Answering queries
If there is no cluster, that contains Z and X together, we need to find cluster with Z and propagate messages along path to clique, containing Z
If we have caching, it can be cheap, because we can recalculate only messages that are along the path
Reference: And more queries
References
- https://www.coursera.org/lecture/probabilistic-graphical-models-2-inference/properties-of-belief-propagation-xm2ul
- https://courses.cs.washington.edu/courses/cse515/11sp/class8-beliefprop-learning.pdf
Cool, isn’t it?
Last update:13 December 2020